$150 million dollars can buy you a lot of things in this world. Maybe you fancy yourself a mansion in Bel Air. Maybe you prefer a private island in Thailand right next to the famous Phuket beach. Somebody, somewhere decided our team at eBay should spend that kind of money on Paid Search traffic, specifically on Product Listing Ads (PLA).
While I might have preferred that private island, access to an unfathomable amount of data was a nice consolation prize. Every year we ran hundreds of experiments and analyzed every inch of our data to find the next growth opportunity. If data is the new oil, you can imagine what kind of insights $150 million dollars can get you.
With billions of visits, you can validate every idea and run every test. We were generating more PLA impressions in a single month than there are humans on earth. Nothing was off the table. We could explore exciting ideas like if stormy days led to higher value clicks (they do). Or we could validate if searches from users located in high-income areas led to a higher initial order size (they did). And we could experiment with exciting ideas like using negative keywords to give us keyword-level bid adjustments in Product Listing Ads (we did).
And while that kind of scale can present some of its own unique problems, it doesn’t mean what we learned can’t be applied to your business. In this article, we’re going to talk about three key lessons that a $100M+ budget can buy you, and how you can apply these learnings to your own paid marketing channels.
We’ll cover these three concepts:
- Marginal ROI
- Incrementality
- Lifetime Value
Marginal ROI (mROI) in Paid Search
Let’s play out a quick scenario for a minute.
You’re the head of marketing for a medium-sized business spending $250,000 per quarter on Paid Search. It’s Monday morning, and you get an email from your CFO:
“Your budgets are increasing. We would like you to spend an additional $25,000 next quarter. You can absorb that kind of budget increase, right?”
You send off a quick email to the paid search team and you get back something like this:
“We had an ROI of +30% last quarter on $150k of spend. ROIs are holding strong, we can take on that increase while staying ROI positive in the channel.”
There’s a major flaw here. ROI means nothing.
Your team is probably right that the overall ROI of the channel will likely stay positive. However, digital marketing is heavily dependent on the law of diminishing returns. It suggests that as you saturate high-performing areas, the incremental revenue per dollar spent will decrease.
Put another way, it doesn’t matter if the ROI of the first $150k is +30%. What matters is what the ROI is on the next $25k which could be. Plus 20%? Maybe negative 50%? But it won’t be more than or equal to 30%.
We firmly believed mROI gave us the best chance to maximize the value of our spend. On a 9-figure budget, every percentage improvement made a difference.
We didn’t just apply this at the channel-level. We would calculate the mROI across every segmentation we could think of. Our goal was to adjust our bids to try and balance the marginal ROI across every key segment to ensure we were making the right investments to maximize efficiency of the channel. We looked at segments like devices, countries, and ad formats (ie: PLA vs. Text).
While mROI sounds complicated, here’s an easy guide to help you do this without some of the overly complicated intricacies.
- Get your daily spend and revenue for each device over some period of time. You’ll segment this on data on a daily basis. Your data should look like this:
Day | Spend | Revenue |
7/1/2024 | $5,592 | $8,295 |
7/2/2024 | $3,661 | $5,152 |
…. | … | … |
2. Forecast your mROI for each device. It often helps to plot in a scatter plot so you can visualize your ‘mROI Curve’.
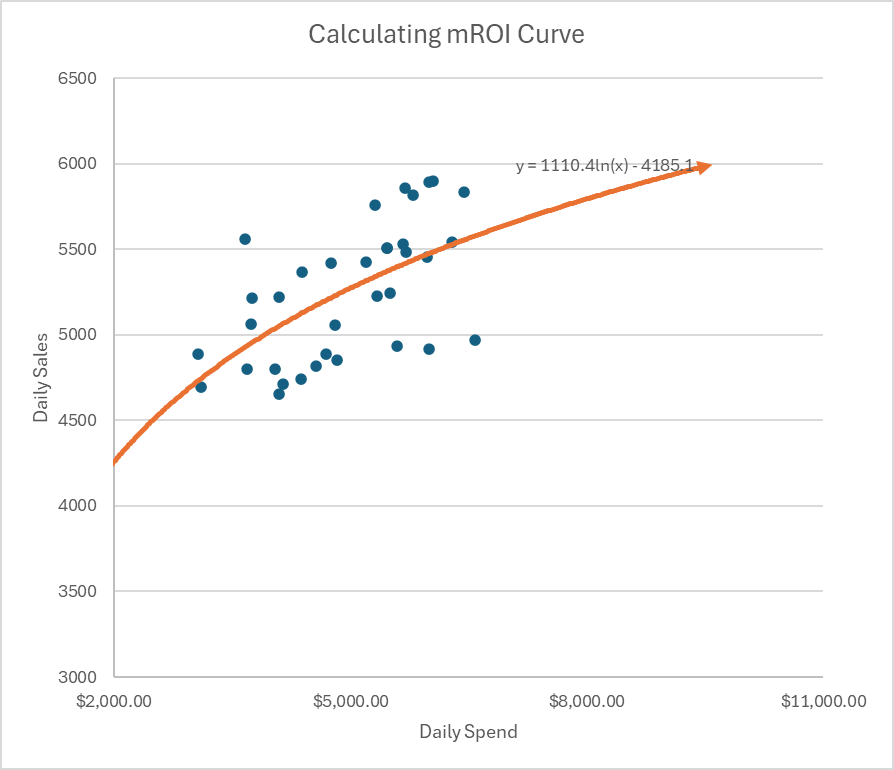
3. Calculate the spend allocation across the Desktop, Mobile and Tablet curves where the mROI is similar.
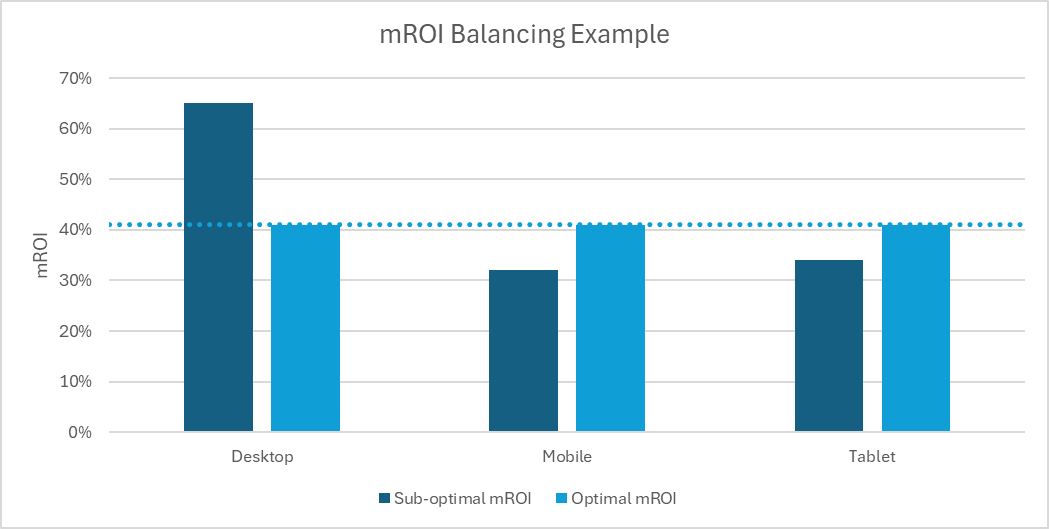
4. Adjust your bid modifiers over the next few weeks to hit your optimal allocation.
When this became too much for our team to do manually, we built machine learning models to automatically forecast the mROIs and adjust bid modifiers programmatically.
Geo-Based Incrementality Testing
Absolutely nobody on this planet likes paying for something they would have been given for free. That’s true with ice cream, t-shirts, and it’s especially true with customers.
Paying Google $14 for a click from a user who would have clicked your big blue free organic link can feel like a punch in the gut. At the same time, it’s an unavoidable problem for any channel in the digital marketing space. Paid search is no exception. Targeting isn’t perfect, attribution is far from perfect, data regulations are getting tighter, browsers are starting to lock down your ability to measure and retarget users.
Despite it being unavoidable, it’s still mission critical. Without that understanding you can’t properly value the overall impact each channel is having on the business. It leads you to over-invest in some channels, under-invest in others, and that lowers the impact you’re having on the business.
Even worse, if you don’t do this you’ll find yourself stuck in another 2-hour meeting discussing what the right attribution model is. That eventually leads to the same conclusion every single year: No attribution model is perfect.
At eBay, incrementality was a big part of our data-driven approach to understand and value each channel as best we could in our overall marketing mix. We didn’t just run these tests one time and forget about them, We ran these tests continually.
Internally they were nicknamed “dipstick” tests. For the uninitiated, a dipstick is a simple tool used to measure the level of liquid in a container, such as the oil or gas in a car engine. They’re built to allow for easy retests because you don’t just check how much gas you have left and forget about it. You check early and often.
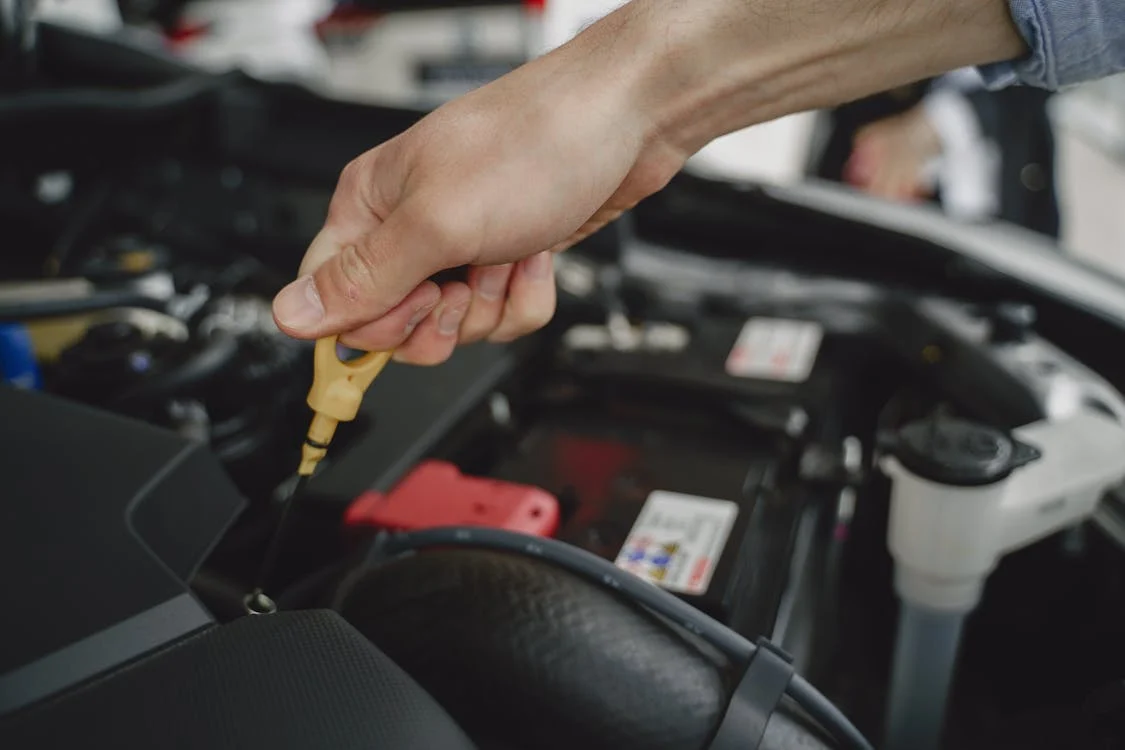
We approached incrementality in a similar way. We found it often changes as market dynamics shift. Channels are constantly evolving and incrementality shifted as our channel strategy evolved. Incrementality is something that needs to be in your plans year-in and year-out.
While there’s many approaches to designing and running incrementality tests we went with a geographic-based incrementality test. This involves turning off the Paid Search channel in certain regions to understand the overall business impact relative to regions where Paid Search remained on. The idea was to create a controlled environment where we could isolate the effect of paid search on overall conversions
Here’s how we did it:
- Identify Comparable Regions: The first step was to identify pairs of regions with similar behaviors in terms of traffic, conversion rates, and overall sales performance. This might mean pairing two metropolitan areas with comparable demographics, or two regions with similar market conditions.
- Measure Noise: Conduct power analysis and stability tests to validate the minimum detectable change for the test. You don’t want to run an incrementality test that requires 6 months to get back statistically significant results.
- Control vs. Test Group: In each pair, one region was designated as the control group where paid search ads continued to run as usual. The other region became the test group where paid search was completely turned off. This allowed us to observe the changes in overall conversion rates and sales performance without the influence of paid search.
- Measure the Impact: Over a set period—typically a few weeks—we closely monitored the performance of both regions. The key metrics included conversion rates, total revenue, and other business KPIs. By comparing the performance of the control and test regions, we could assess the true incremental value of paid search.
- Analyze and Adjust: Once the test concluded, we analyzed the data. If the test region (without paid search) saw a significant drop in conversions, it indicated that paid search was driving incremental value. Conversely, if the impact was minimal, it suggested that a portion of the budget might be better allocated elsewhere. Typically this was expressed as a percentage of total sales.
- Iterate and Evolve: As market dynamics shifted, so did the results of our dipstick tests. That’s why these tests were not a one-and-done exercise. We repeated them regularly, adjusting our channel strategies based on the latest insights.
There are a few important warnings on running incrementality tests:
- Incrementality tests can be tricky without the right analytics partners to help design and analyze the data. Business decisions on bad data are far worse than decisions on no data at all.
- Not every business is suited to running incrementality tests. The smaller your channel’s contribution to the overall business, the more conversions you need for a statistically significant result. You can use statistical methods like a power analysis to help understand what’s possible and how long the test will take.
Warning aside, if it’s not possible to partner with an analytics team you’re not stuck. LLMs like GPT can be a powerful tool to help anybody work through designing and analyzing an incrementality test if absolutely needed.
Customer Lifetime Value (LTV)
Over the last 10 years, Google has slowly made more and more changes to try and make advertisers’ lives easier. They introduced variant-match keywords to reduce the burden of finding and managing keywords. They introduced auto-bidding models to brands bid smarter and more effective. They’ve rolled out entirely new campaign types without any keywords to manage, and they’re automatically generating ad creatives now.
They’ve done a lot of things to try and help advertisers but the downside to all of that is the differentiation between you and your competitors is slowly shrinking. As Google continues to automate more and more of the PPC function, the biggest differentiator you’ll control as a paid search manager is your data. And the most important data you have is your conversion data – Who is converting, and how much are those customers worth.
Customer worth, aka Customer lifetime value, is calculated using historical data. Businesses like eBay look at things like “How many orders did the average customer have in their first N months as a customer?” to start to understand the total value they get from the customer. They’ll often group these customers by the year of their first purchase so they can understand how LTV has changed over time, and there are often entire teams responsible for improving the LTV of their customers.
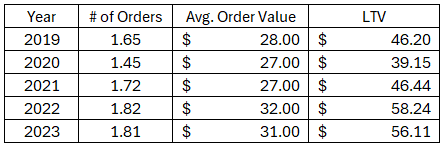
While some brands choose 12 months, others might choose longer windows to capture all the potential future value from that customer.
The window you choose has meaningful repercussions:
- You want a long enough window to accurately capture the expected value that a customer will generate over their lifetime. If a customer spends the next 5 years using your service, a 1-year window will undervalue the contributions of your channel.
- You want a short enough window to measure the change in LTV in a timely manner. For businesses that use a 5 year-window, they need to wait a full 5 years to get a full picture of how their customer LTV has shifted.
While I won’t share what LTV window eBay used during my tenure, I will share a few interesting insights from my time there.
- For one thing, there are other segmentations that matter. For example, the first product a customer bought had a material impact on their overall LTV. Dimensions like product category, and product price played an important role in determining their expected customer lifetime value. Customers who bought expensive items with their first purchase tend to drive more future value as well.
- “Future Value” was a meaningful allocation of our total value generated from our channel. When experimenting with different bidding and grouping models, that allocation shift was an important part of our discussion.
- You can get creative with mining ways to extract and measure value. We explored the concept of the “halo effect” of a new customer in a household and how it helped us capture new customers within the same household. The better you can get at finding ways to quantify value of your customers, the bigger the advantage you have over your competitors.
As more of the market adopts these paid models (the same models you and your competitors are using) the difference between success and failure is largely your ability to understand the full value of each new customer and communicate that back to Google. It’s the companies with the best conversion signals and conversion data that win the PPC war. Not just the order value of that first conversion, but also the total added value that customer creates for the business.
- How many additional purchases will that customer make with my business? What are my margins on those additional products?
- Are we turning those customers into brand ambassadors? How many new customers are they creating for our business? Can we measure that too?
- What was the marketing value of the initial conversion to our brand now that we can communicate and retarget this customer? How does the email marketing team value each new email address? How does the display team value the ability to retarget all of our incremental paid search traffic? Talk to your marketing partners. Figure out where you’re adding value. Take credit for it, and feed that back into Google.
If you’re not calculating customer lifetime value, here’s the simple way to approach it.
- Looking Window: Pick a reasonable lookback window for your LTV calculation. If you’re unsure, a 1-year window is often a safe choice.
- Calculate Customer Value: Find your customers’ first purchase date and add up the order value of all of their orders within 1-year of that period.
- Customer Grouping: Depending on your data volume and business need, you’ll be able to group based on week, month or year. You might need to even group multiple years together if your conversion volumes are too small.
- Average The Values: For each group of customers, calculate the average value. I always recommend computing the median value as well so you’re aware of a small handful of outlier customers who might be skewing the typical customer value you’ll be expecting from Paid Search.
Conclusions
The strategies and insights we’ve discussed—Marginal ROI, Incrementality, and Customer Lifetime Value—are powerful tools for any marketer, regardless of budget size. The key takeaway is that success in paid search hinges on a deep understanding of how each dollar contributes to your overall business goals.
By focusing on the incremental value of your spend, rigorously testing your assumptions, and understanding the long-term value of your customers, you can make informed decisions that drive meaningful results. These principles are not just for large-scale campaigns; they can be applied to campaigns of any size to optimize performance and maximize return.
As digital marketing continues to evolve, your ability to leverage data and adapt to changing dynamics will set you apart. Whether you’re running a small business or managing a larger budget, these lessons can help you make smarter decisions and achieve better outcomes in your paid search efforts.
Disclaimer: The views and experiences shared in this article are the author’s own and do not reflect the official positions or opinions of eBay. All information is based on personal experiences during the author’s tenure at eBay and should be considered in that context.